Game Theory is a term that is used liberally in the Procurement profession at present. It’s often used in the wrong context and is in danger of being regarded as a fad because it has been oversold as a panacea. It is quite common to see new ‘Centres of Excellence’ for Sourcing and Negotiations springing up with the intention that Game Theory is central to their strategy. Whilst Game Theory offers some important learnings, to drive tangible benefits a plan for how excellence is adopted at scale is the main decider for success. Credible plans to scale excellence are less common than organisational plans to develop a Centre of Excellence. Building a strong core team is the easy part; delivering value across large organisations into the hands of colleagues in far-flung countries is the real challenge. Can Game Theory really help so these new Centre’s of Excellence deliver on their potential? As somebody with a PhD focused on Game Theory in auction settings, I argue in this post that traditional methods in Game Theory have been oversold and scalable approaches leveraging Algorithmic Game Theoryand Algorithmic Mechanism Design offer scalable solutions that enterprises require for the kind of results they are seeking. Game Theory can act as a guide for intelligent systems that automate best practice at scale. This is the key to unlocking potential so we break down our analysis of how Centre’s of Excellence need to merge business strategy, Game Theory and technology strategy as follows.
- Economists lost interest in Game Theory and then rediscovered an interest when the internet injected new momentum. We explain why this is so.
- What is Game Theory exactly? We touch on the core principles that can guide procurement leaders in terms of what it is useful for and where its limits are reached.
- How is Algorithmic Game Theory different and what is the relationship with Algorithmic Mechanism Design.
- From Poker Bots to Sourcing Bots — why the recent advances in Poker Bots are instructive for Procurement.
- Conclusions on Game Theory and the Future of Procurement.
The Internet Age and Game Theory
Game Theory can be useful as a model of human decision making in a strategic setting that requires some assumptions about how decisions are made. Alone, it’s simply an explainer (or normative) discipline that is scientifically interesting as it guides expected behaviours. However, on its own it doesn’t deliver solutions for grand challenges facing large enterprises that require real solutions in a timely fashion. The internet has heralded an age where automation of decision making means that software agents can encode rationality thus making it more predictable. When negotiations are operated by Bots, efficient outcomes can be systematically discovered faster and it can drive truly transformational changes. So the advent of intelligent software systems to automate processes and decision making is a key factor in the re-emergence of Game Theory as an important field. When economic bargaining (typically via a bidding mechanism) is conducted by software agents then the scientific methods for designing these agents are informed by Game Theory, Artificial Intelligence (AI) and Operations Research (OR). The notion that a Centre of Excellence with experts in Game Theory can guide large teams of Sourcing Managers in best practice techniques to execute best practice is fanciful. The technology giants of Google, Facebook and Amazon are not aiming to educate large numbers of staff on cutting edge techniques; instead, the strategy is to have a strong core team building intelligent systems that can implement best practice at scale. This is the path that Procurement in large enterprises needs to follow. But first, it needs systems that are designed to support intelligent automation that leans on Game Theory and AI and OR.
Game Theory Assumptions narrow the Field of Applicability
It is worthwhile taking a recap on what Game Theory is because it is often misunderstood. We start with the foundation stone for Game Theory that the renowned Prof John Nash recognised as a result that could explain why ‘equilibria’, or solutions to games, always exist. Many people question the following mathematical pillar when they hear it because it sounds implausible. Brouwer’s Fixed Point Theorem is quite remarkable and may be understood in an example as follows. Take two sheets of A4 paper, one lying directly above the other. If you crumple the top sheet and place it on top of the other sheet, then there must be at least one point on the top sheet that is directly above the corresponding point on the bottom sheet. It doesn’t matter how crumpled it can be as long as it’s not ripped. Or another way of explaining is if you are standing in a city, say Amsterdam, and you have a map of Amsterdam on the floor then at least one point on the map is directly above the point in Amsterdam it refers to, even if you crumple up the map. And it gets even more mind-bending. In three dimensional space, Brouwer’s Theorem says that if you take a glass of water, and swirl it around, then after it settles there is some point in the water which is in the exact location that it was before the swirling. Moreover, if you tried to swirl that point out of its original position, you cannot help but move another point back into its original position.
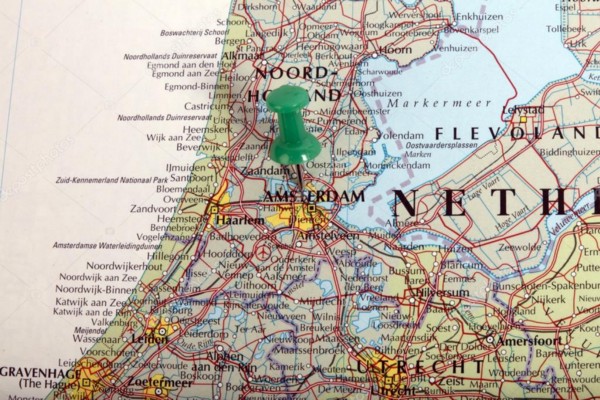
John Nash made the first connection between strategic decision making and this result. In a ’Normal Form’ game, that he studied in terms of a Poker example, each player has a set of strategies to choose from. With two players it is a two-dimensional matrix with strategies listed on the axes. The reward each player receives depends upon the choice made by themselves and the other player. So the key to choosing your best strategy is to understand your opponents best strategy. But she is also making a decision based on an expectation of your choice and this is a recursive loop. So just as there is at least one equilibrium solution in the map or liquid above there is always at least one solution, a Nash Equilibrium, where all players are choosing a strategy that they don’t have an incentive to change. So if we can calculate the Nash Equilibrium of a game, then we can predict expected outcomes. This was the first time that it was possible to build mathematical models of strategic interactions between players with self-interested motives. Nash had envisaged simpler games such as Poker but scientists began a whole new field of research that expanded to include increasingly complex models of games that included Central Bank interest rate decisions, military strategy and most crucially for Procurement, auctions in their most general sense (that include tenders and multi-round RfQs).
Limitations of Game Theory
It’s useful to remember the aphorism that all models are wrong but some are useful. Game Theory as a model is useful if the players are rational, have expectations over their payoff structures and the payoffs of other players for possible outcomes. The assumption of rational decision making breaks down when people make emotional decisions that can override judgement or make decisions that benefit others. For example, Game Theory would predict that people never make private charitable donations. Another limitation is that the model requires prior beliefs for players on at least the probabilities of payoffs for other players. In the absence of such information, it is impossible to model likely outcomes in events. For example, 5G auctions in Germany are closing and trying to apply Game Theory to understand if there will be competitive bidding in the final stages requires better knowledge of the true private values ascribed to the licences by competing companies.
If we have that data then we could model the likely events but in practice, this isn’t usually practicable because the information is not known. Another less discussed but equally serious limitation is computational complexity. The difficulty in computing equilibria in complex environments, even for high powered servers, should not be underestimated. There is a trade-off between how accurate a model is and how difficult it is to compute. Yet another limitation can be that some types of games have a large (or even infinite) number of equilibria and discerning which outcome is most likely could be impossible. So the entire field is fraught with challenges. However, with the advent of the internet, some of these challenges receded because if you are building economic agents to bid in auctions or manage sourcing events then you can encode rational behaviour in the Bot. The explosion in data has also aided modelling of players potential beliefs and the advances in computing power and algorithms have meant that previously inscrutable problems are now within reach.
Algorithmic Game Theory — Industrial Procurement needs to learn from Tech Giants
In the last fifteen years, Game Theory has enjoyed a rebirth due to two key factors. Game Theory predicts the actions of software-based agents more reliably because bots bidding in auctions or algorithmic trading agents in financial markets tend to be encoded to act rationally and are thus more predictable. Secondly, the explosion in computing power and data storage has allowed previously intractable models to be solved or approximately solved quickly. Internet giants such as Google, eBay and Amazon recognised early on that to attain fast growth rates they needed economic mechanisms that scaled quickly and performed as well as or better than human experts. Hal Varian’s first auction design for sponsored search results at Google was a milestone, not so much in terms of economic theory, but because it was a large corporation accepting that a fundamentally different model to scale excellence is required. Bidders competing in the auctions to present adverts to users were equipped with a tool to bid according to rules they could control. Crucially, the amount they paid for an advert to appear or be clicked on was capped at their bid price and not necessarily the amount they paid.
The payment rule wasn’t the simple rule applied in almost all RfQ’s or reverse auctions we see in Procurement where suppliers pay the amount they bid. Algorithmic approaches compute a payment amount that they could have bid (a lower value if they were actively monitoring competitors bids) and charge a payment calculated by an algorithm that is loosely based upon the second price. All auctions (a tender or RfQ is a type of auction) comprise a payment rule and an allocation rule. An efficient auction doesn’t necessarily award the items to the highest bidder. If you’re thinking long term like Google was then you want higher quality adverts (with higher clicks per viewer) so Google also devised an algorithm for ranking the order of adverts to instil higher value for their users. This was transformational for Google, their users and advertisers because it allowed bidders to instruct a bot to act on their behalf and walk away knowing they would have acted the same if they were watching the bidding and their time was invested in their core service. Users benefited because higher quality adverts rose to the top. Advertisers moved in droves to Google’s platform, users moved in droves too and other search engines lost out. In the macro game of dominating search, Google had won largely thanks to Algorithmic Mechanism Design. This was their secret weapon.
Most Procurement teams aren’t yet ready to jump on board with this approach fully because they are using legacy systems that don’t store data in a form that can enable AI to automate upstream processes. In the words of Mark Twain, ‘the best way to get ahead is to get started’, so commencing Sourcing Automation on targeted categories so that you begin the process of encoding your upstream processes as soon as possible. Sourcing Bots can be category specific software agents that embed category intelligence or they could be general systems that execute more basic RfP or Auction protocols when called upon. Both approaches can apply Algorithmic Game Theory by starting with a fixed sourcing process and then allowing the system to explore slight changes and learning over time what strategies in these games work best. This type of autonomous system can cautiously explore a strategy space faster than humans and learn across global organisations. The approach can move from category to category over time so that legacy systems eventually become redundant for sourcing and then even some downstream systems also become redundant. For example, if all contracts generated by Sourcing Bots are structured software objects, then contract analytics is no longer required for those auto-generated contracts.
From Poker Bots to Sourcing Bots
Whilst the study of behaviour in games is referred to as Game Theory, the study of how you should design the rules of a game to get players to behave in the way you wish is Mechanism Design (or Inverse Game Theory). Algorithmic Game Theory is the study of behaviour in complex environments (usually internet settings) and is a loosely used term to cover Mechanism Design also. This technology grew rapidly in many settings with tech giants leading the charge. Amazon applied intelligent pricing algorithms and released recommender systems to cross-sell. Facebook devised intelligent connection recommendations, applied similar auction mechanisms for adverts and scaled revenue growth via intelligent systems that employed Algorithmic Mechanism Design. So Procurement professionals, strictly speaking, should be talking about applying Mechanism Design to enhance performance rather than Game Theory. It is your suppliers that apply Game Theory to analyze their bidding and tailor their strategy in response to the sourcing event (the mechanism) they encounter.
Its deployment in Procurement has more obstacles remaining but they are receding. Most legacy systems can be considered an obstacle because they fail at the first hurdle. Namely, the data is not stored in structured tables, doesn’t undergo rigorous (automated) cleaning and isn’t normalised. Crucially, clean and normalised data is the precursor to infrastructure for automation of procurement. Sourcing systems are the inlet valve for new data from suppliers so it is at this ‘upstream’ point in the process that it is vital to begin the process of gathering clean normalised data. By normalised, we mean data whose semantics are understood and by clean we mean we can be confident that the values are sensible and not polluted with noise or errors and thus calculations can be made with confidence. The automated execution of best practice sourcing processes is then possible but relies upon a Sourcing Optimisation system to gather expressive information from suppliers and compute optimal outcomes that incorporate cost and non-cost objectives as well as constraints and preferences. Sourcing Optimisation is the most flexible sourcing technology available and also supports data storage requirements so its the engine that Sourcing Automation relies upon to automatically execute events, learn from them and then enhance the Mechanism over time. This loop of Machine Learning coupled with automated execution is the virtuous circle to feed autonomous procurement systems that can become the equivalent of robotic Chess Grand Masters or World Poker Champions.
The Future for Procurement
Just as sponsored search terms, e.g. “lawyers in Chicago” or “cobalt suppliers in South America”, were commoditised and auctioned with weighting given to higher quality bidders that tended to receive more clicks, so too will many goods and services become increasingly commoditized as standards impose competition across many industries. And similarly, the processes for sourcing these can become automated because they are predictable. Furthermore, that automation will extend to suppliers responses to sourcing events. Fully automated negotiations will become common and the spend thresholds for which they are automated will gradually increase over time. This will not be the case for all spend categories, but for some of the big ticket spend items there are competitive markets (eg. Logistics, packaging, materials, IT services, Infrastructure as a Service, facilities management, hotels, travel, car rental, steel etc).
But during this evolution, it’s important to remember that Game Theory is more of a guide to best practice than a silver bullet. Engineered Systems that apply Game Theory, Machine Learning, Statistics and expert knowledge are being combined in solutions that empirically test and continuously improve over time as automation becomes increasingly common. This is happening already and Keelvar is leading a new field of Sourcing Automation that is applying AI and Game Theory to scale excellence for the world’s leading procurement teams.
— — — — — — — -
Author: Dr Alan Holland is the Founder and CEO of Keelvar. He was previously a Lecturer and Research Fellow in University College Cork where he specialised in AI and Game Theory.
Learn more how Sourcing Bots are developed and operate at Keelvar Konnect 2019: https://konnect.keelvar.com
Relevant resources: Sourcing Automation and Sourcing Optimization